Three decades ago, the introduction of electronics into vehicles marked a significant shift in the automotive industry, requiring new skills and working methods. The arrival of active safety functions, automated driving features, and digital cockpits accelerated this trend. The advent of over-the-air (OTA) updates ushered in a new era, allowing vehicles to evolve after market release, integrating new functions and services customers would pay for. This is the era of the software-defined vehicle, where software activities are primarily limited to functions within the vehicle.
The landscape further evolved with OpenAI’s release of ChatGPT in 2023. This development made industries realise the potential of generative AI and the impact that new applications and services would have on their businesses. Capgemini estimates that 16% of large automotive OEMs can increase operating profits by deploying AI at scale.
AI will not only improve functions within the vehicle but will also enhance efficiencies across the automotive production workflow. That includes anything from designers using AI-generated images to imagine new vehicles, to design and engineering teams using generative AI application programming interfaces to connect their tools to build digital twins of their facilities, to marketing and retail sales departments adopting generative AI tools to brainstorm and develop marketing copy and advertising campaigns.
Most automakers are evaluating 100 to 150 use cases for AI deployment, making it hard to imagine a single function that will not be improved with generative AI. This transformation leads to a new era, the “AI-defined automaker.”
Knowledge chatbots enhancing customer service
Ford, which receives around 15,000 customer calls a day, uses generative AI throughout the call centre workflow. First, a speech-to-text system automatically manages the data associated with the customer request while removing any personal identification information, which is mandatory in many countries. This intelligent data management enables the creation of a second AI service, a chatbot used by supervisors and engineers to query the database on questions such as the number of call centre calls or even how many were related to a specific issue. Additionally, AI assists the call centre operator by suggesting the best answers to customer queries, thereby improving call centre speed, efficiency, and customer satisfaction.

Another example is the use of knowledge chatbots at repair shops and dealerships. For example, if a vehicle’s air conditioning is broken, the technician can simply ask for the best way to repair it on the specific car model, and the chatbot will provide step-by-step instructions and visual examples.
Automated vehicles (AV) 2.0
AV 1.0, the traditional way of developing automated driving functions, is based on a modular architecture of perception, prediction, and actuation. Perception is primarily based on Convolutional Neural Network (CNN) classification AI, and it was a common belief that by solving perception, meaning having perfect classification of objects in a driving environment, the autonomous driving problem, even for high levels of automation, would be solved. However, after more than a decade of efforts, it became clear that the long tail of use cases is endless, and more than perfect perception, a right interpretation of the scene is required for the vehicle to manage previously unseen scenarios.
Transformer engines, which are the foundation of generative AI, are not trained exclusively on automotive data but on general data, enabling them to abstract and correctly understand driving scenes that have never been encountered before, making the right decisions. This is the core of AV 2.0, where an end-to-end architecture, with sensors’ raw data as input and vehicle actuation (acceleration and direction) as output, replaces the traditional AV 1.0 modular architecture. In addition to performance benefits, this approach optimises the computational resources allocation, as there is only one function being calculated, rather than several modules competing for available resources. It also facilitates the transfer of a vehicle to different cities and countries, a difficult problem for rule-based AV 1.0 systems.
Startups like Wayve, a developer of embodied AI for assisted and automated driving, and Waabi, an AI company building the next generation of self-driving technology, are making tremendous progress in this field, demonstrating impressive results.
The superpower of digital twins
Digital twins are physically accurate virtual replicas of assets, processes, or environments that exist in the physical world. In industry, digital twins can be used throughout the product lifecycle, from design and manufacturing to maintenance and operations, to sales and marketing.
Digital twins enable physically accurate visualisation and precise testing of different product versions and scenarios. They allow for superpowers: travel in space to virtual factories or inside vehicle prototypes to help teams optimise designs, and travel through time to understand past errors and avoid future ones by working on alternative futures. Instead of teams relying on in-person meetings and static planning documents for project alignment, digital twins streamline communication and ensure that critical design decisions are informed by the most current data. Digital twins allow project stakeholders to visualise designs in context during design. Teams can identify errors and incorporate feedback early in the review process.
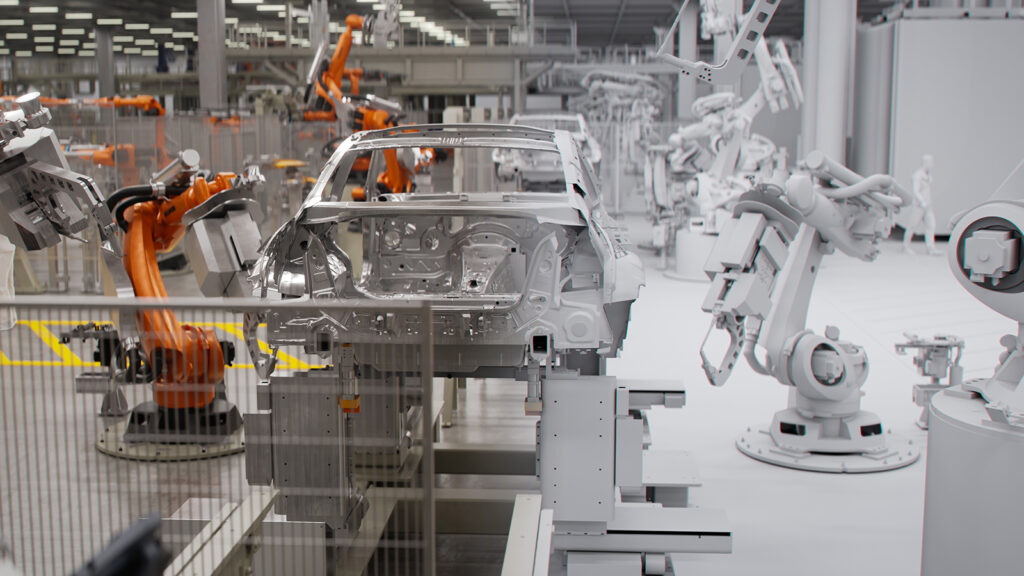
Recent advancements in digital twins include the adoption of OpenUSD (Universal Scene Description), an extensible framework and ecosystem for describing, composing, simulating, and collaboratively navigating and constructing 3D scenes. HTML is the standard language for the 2D web, while USD can be seen as the powerful, extensible, and open language for the 3D web, enabling communication between the many different tools and siloed departments in the product life cycle. The latest developments in physically-based rendering and accelerated scalable computing enable the creation of large-scale, physics-based digital twins that are truly representative of the real world.
In early 2022, BMW introduced the iFACTORY for virtual planning in digital twins. All of the BMW Group’s vehicle and engine plants were 3D-scanned, as well as more than seven million square meters of indoor and 15 million square meters of outdoor production space. BMW has a vast workforce of factory planners, including those working at facilities like the future Debrecen plant, who play a critical role in the planning and operations of the company’s factories worldwide. Their jobs are highly complex, and even the slightest miscalculations or mistakes can result in massive real-world costs.
This is why physically accurate virtual planning is highly desirable, as it allows planners to experiment and make changes at practically no cost. Planners can pre-optimise production processes using virtual environments before committing to massive construction projects and capital expenditures. This approach significantly reduces costs and production downtime caused by change orders and flow re-optimisations on existing facilities.
The combination of digital twins and generative AI further enhances the benefits of each. Generative AI can assist in generating assets, providing teams and customers the ability to interact with assets and knowledge bases in natural language, navigating the digital-twin environment, and creating visually and physically accurate digital worlds for product development and training, such as teaching autonomous vehicles to drive or robots to walk. The digital twin becomes a digital gym or training ground in which the product’s software functions can be developed. Digital twins are also used for synthetic data generation, creating the data required to train the AI algorithms that will be used by the product being developed.
The AI-defined automaker is here today
The automotive industry is undergoing a profound transformation, not only introducing software-defined vehicles, but also entering the era of the AI-defined automaker. Generative AI is revolutionizing every aspect of the business, including design, engineering, customer service and autonomous driving. Empowered by advancements in digital twins, automakers are rapidly deploying AI-powered solutions to boost efficiency, innovation, and customer experience. As the industry embraces this AI-driven future, every function can be enhanced and optimised by the power of generative AI. This evolution promises to unlock new levels of efficiency, innovation, and customer-centricity, positioning the automotive industry for continued success in the years to come.
About the author: Pedro Moreno Lahore is Senior Account Executive, Automotive, at Nvidia