In today’s world, disruptive technologies such as artificial intelligence (AI) and IoT are transforming automotive operations. Global automotive giants such as Toyota, Jaguar Land Rover, and Ford are already leveraging automation to replace manual inspection processes throughout the finished vehicle supply chain. These companies are embracing this as part of Industry 4.0: utilising automation, machine learning, and real-time data to drive significant business benefits. The outdated manual processes of traditional vehicle inspections‚—which are not only expensive but also time-consuming—are now being replaced by advanced automation processes to simultaneously improve performance, drive efficiency, and reduce costs.
The role of AI and machine learning in automating vehicle inspection
AI and machine learning have a long history, but it is only very recently that hardware and software advances have made automated vehicle inspection solutions commercially feasible. The recent combination of lightweight multithreading (large numbers of cores that are organised in groups to parallel process workstreams) enabled by advances in graphical processing units (GPUs), combined with frameworks for convolutional neural networks (CNNs), and ready access to powerful cloud computing platforms to process millions of images, allows companies to accurately detect defects on a vehicle and provide the data in a matter of seconds.
Artificial neural networks replicate how human brains work; nodes are connected through a network of weighted links, equivalent to the biological nodes in the brain that are connected by synapses. Whereas in conventional computing data is stored in bytes, 0 or 1, and modified through Boolean operators (e.g., addition, subtraction, etc), for neural networks it is the weighting of each link between two nodes that is important. A neural network has multiple nodes, creating a pattern of links between them, that allows even extremely complex characteristics to be modelled.

Therefore, by feeding vast amounts of previously seen and annotated data, these characteristics can be modelled by the neural network, and in turn, used to understand whether the same characteristics are present in new unseen data. These techniques have been used very effectively in medicine to train neural networks to recognise diseases and in turn process large datasets that no human could reliably or effectively make sense of, diagnosing patients far more accurately and consistently than previously possible.
GPUs are ideally suited to speeding up this process as they perform vast numbers of multiple, simultaneous computations, unlike traditional computer chips, to model the links in the neural network. Access to scalable computing power in the cloud provides the third component: the means to process and store huge amounts of data without needing to build expensive in-house or on-premises infrastructure.
Accurately locating where this damage occurs in the value chain allows for correct liability assignment—the prerequisite for process improvement
These technological advances now make it possible to inspect millions of vehicle images and accurately classify even the smallest defects. Dents, dings, scratches, and chips that would often be overlooked by an inspector can be identified and digitally displayed on a dashboard in less than a minute. Consistent results are achieved in each and every location and inspection, a huge benefit for an OEM looking to standardise quality across a global supply chain. These visible damages are a significant cause of warranty costs for OEMs and in-transit damage liability for logistics providers. Accurately locating where this damage occurs in the value chain allows for correct liability assignment—the prerequisite for process improvement.
The benefits do not stop there. Trained algorithms are also able to identify specification errors in the vehicle assembly process. Incorrect parts or even missed components, such as spoilers, wheels and badges can be flagged in seconds, allowing for rework before a vehicle leaves the factory.
Strengthening AI with high-resolution images
Computers need to be able to see and interpret images as an array of pixels. The resolution of the vehicle image is therefore critical in determining the accuracy of defect detection. In short, image resolution is everything. Due to the contrast in shadows and highlights across a highly reflective surface, a sensor with a wide dynamic range, or gamut, is also critical to ensure a high-quality image. And once again, technical advances have made this possible. Sony, for instance, provides the most advanced, large format, digital sensors on the market, capable of identifying scratches as fine as human hair and paint chips as small as 1mm in diameter. These sensors are also extremely durable, with a much higher resolution and wider gamut than conventional machine vision cameras.
Lighting is the other key factor necessary for the highest resolution images. Automated vehicle inspection specialist DeGould, for example, deploys a patented combination of three different light fields in the Auto-compact system: white light, dark light, and banded structured light, which are specifically designed to cater for the different spectral properties of the various defect types. For example, many scratches and dents will transition between highly visible and entirely non-visible depending on the vehicle colour, light flux, and spectral properties of the body panel.
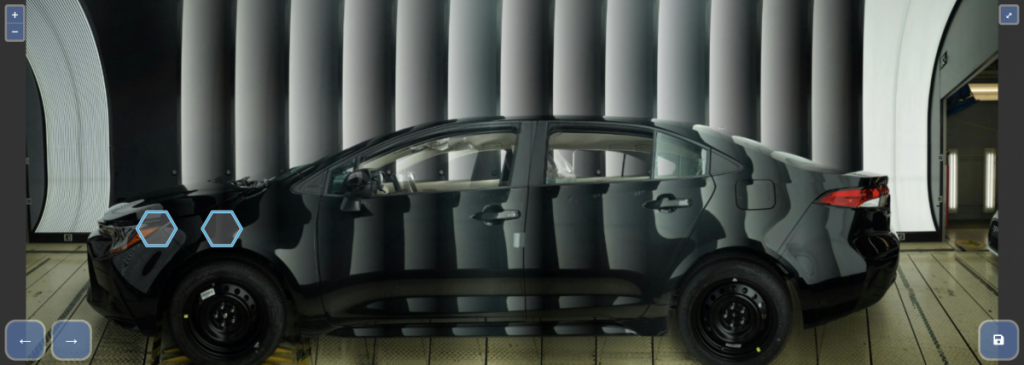
The future of vehicle inspection
The real game-changing opportunity for OEMs today is to deploy AI throughout the finished vehicle logistics chain. By automating inspections from the production plant and at each handover point until the vehicle arrives at the dealer, the process can be transformed. Improved accuracy can be combined with significant cost-saving opportunities.
Technology advances have made this transformation both feasible and cost-effective. By digitising this entire process, a unique digital vehicle passport can be created, providing an accurate record of the vehicle’s condition throughout its journey. All partners in the chain will benefit from correct, consistent and reliable damage liability assignment—the essential first step for identifying process improvements and cost avoidance. Automation also allows for some, but certainly not all, human inspectors to be redeployed to higher value-add functions within the business.
About the author: Benjamin Jones is Head of Machine Learning Strategy at DeGould