Autonomous vehicles are already here, with trucking and fleets leading the way. Autonomous trucks, operating at least at SAE Level 4 are plying the roads in Texas, California and elsewhere, and driverless buses are on the way. While self-driving passenger vehicles are slightly behind, they will be here—en masse— very soon, experts say.
Who or what is at fault?
And while many believe autonomous vehicles will result in greater road safety, no technology is perfect. Even with their currently limited numbers on the road, self-driving vehicles have been involved in collisions and can, just like any other vehicles, sustain physical damage, even from minor incidents. As more autonomous vehicles take to the road, the number of incidents in which they are involved will grow. Questions will arise about the viability of the vehicles themselves, as well as about the quality of their software or the control systems that guide them.
And those questions will be exacerbated by the fact that autonomous vehicles will be sharing the road with driver-controlled vehicles, pedestrians, bikes, motorcycles, electric scooters perhaps even futuristic hoverboards. At that point, the lawyers will go to work; attorneys, courts, and owners will have to grapple with issues of responsibility. But to determine responsibility for an accident—ie, who pays—they will have to delve deeply into the different “responsible parties” that may have caused it.
The ideal inspection for autonomous vehicles combines the deep analysis capabilities of AI systems with human supervision
Was there a problem with the vehicle’s on-board software or with the transmission of instructions from the central server? Did the vehicle owner fail to apply a mandatory software update? Was the problem with the vehicle itself, with a flaw developing because of a manufacturing issue? Was the incident due to a problem in the 5G communication network on which autonomous vehicles will rely? Was it due to nothing more than a flat tyre, and if so, did the owner fail to inflate the tyre properly?
Analysis
The only way to reveal the answers to these questions is with a deep-dive analysis of all aspects of the vehicle—both physical and software-related—using advanced technologies like artificial intelligence and machine learning, as part of a general inspection and condition report. While inspections are standard for driver-controlled vehicles, they will play a far greater role for autonomous vehicles, because the responsibility for vehicle and road safety goes beyond just the driver. And AI systems are the most efficient way of conducting these inspections.
These legal issues are already manifesting; adding to Elon Musk’s recent problems is a criminal investigation of Tesla over crashes of vehicles using its semi-autonomous driving software. The Department of Justice is investigating whether the company misled owners into believing that vehicles were “more autonomous”—that they could function properly with less driver supervision—than they really are, leading to more than a dozen crashes. This is just one example of a wide array of complicated cases—concerning dozens of issues, from manufacturing flaws to software problems to owner negligence—in which autonomous vehicles are likely to be involved over the coming years.
There are several steps that can be taken to meet the emerging legal challenges, both in advance of an accident and after one. In order to be licensed as fit for the road, many states require vehicles to be inspected—and inspections of autonomous vehicles need to be more advanced than inspections for standard vehicles. Those advanced inspections need to analyse not only the physical integrity of vehicles, but also the integrity of the software running them, both on-board and external.
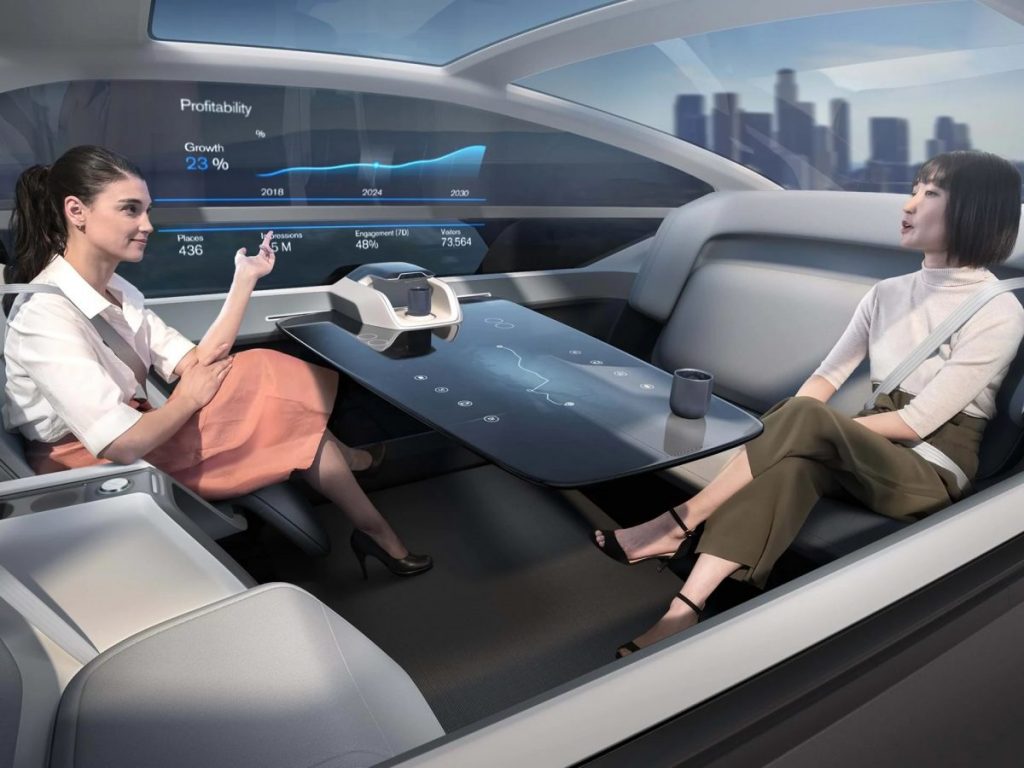
The inspection needs to analyse how the vehicle will act under specific traffic conditions, and compare those situations to a database of previous accidents to determine if a vehicle is in danger of becoming entangled in an accident. In order to accomplish this, inspectors need to adopt AI and machine learning-based analysis systems, which can determine relationships between vehicle condition, software, and road conditions far more accurately than any human inspector could, because of the huge number of variables that need to be checked.
If a vehicle is involved in an incident, AI and computer vision systems can also be used to determine the level of responsibility for each element. By examining the scene of the incident and the circumstances surrounding it—level of traffic, weather, time of day—the system can determine if the software took into account all the factors it was supposed to in order to ensure safe driving. If the software was operating properly, the system can check the integrity of the vehicle—whether all the parts were operating properly or if the vehicle was properly maintained—as well as any possible role played by the human driver, passengers or controllers, or any other external factor. Again, no human inspector could be expected to reach this level of detail in their inspection.
With that, AI inspection systems, just like the autonomous driving system, need to be supervised. While AI systems have significantly reduced the problem of false positives and significantly streamlines the process of decision-making for many organisations, it’s not perfect. And when AI does fail, it tends to fail in a big way. Human supervisors need to monitor AI decision-making to ensure that those decisions make sense—that they conform with the law, that they do not entail undue financial risks, that they do not violate the sensibilities of the public.
Those bad decisions could be the result of numerous factors, from bad programming to bad data. AI problems are difficult to troubleshoot, and with lives at stake, managers of autonomous vehicle grids need to ensure that the system works properly at all times. Until AI systems are advanced enough to diagnose themselves for errors on the fly, human supervision is the best method to ensure autonomous vehicle road safety.
And while AI systems will likely do a thorough inspection job when it comes to the major systems in a vehicle—ignition, motor, braking, and others—it may miss some of the smaller issues that could be just as crucial to road safety. For example, current machine vision systems could “pass” a headlight on inspection, but if the casing of the light is dirty or dusty, it could lose lumen power, making it less bright to oncoming vehicles at night and thus more prone to accidents. The same goes for issues like scratches on a tyre, which don’t affect the tyre’s performance right now, but could quickly cause a deterioration of quality. Human eyes are much more likely to pick up on issues like these, again demonstrating that the ideal inspection for autonomous vehicles combines the deep analysis capabilities of AI systems with human supervision.
Autonomous vehicles and driver-controlled vehicles serve the same purpose, but unlike with the latter, where much of the responsibility for road behaviour lies with the driver, autonomous vehicles are controlled by a variety of factors: software, data networks, OEMs, control centres, the physical condition of a vehicle, and more. So who, or what, is responsible for an accident? Who pays? AI is going to be an important factor in determining the answer to that question.
About the author: Neil Alliston is Executive Vice President of Product & Strategy at Ravin.ai