Advanced driver assistance systems (ADAS) have progressed considerably in recent years. Blind spot detection, lane assistance, and collision avoidance are just a few of the systems closing the technological gap on the road towards true vehicular autonomy. But to borrow a quote from our impatient backseat passengers, “Are we there yet?” Not quite. There are still roadblocks between where the industry stands and where it’s trying to go. But each obstacle the automotive industry encounters is a catalyst for innovation, bringing us closer to an era of mobility in which autonomous driving is not just ubiquitous, but incredibly safe.
The issue often boils down to the software models that power ADAS applications. These deep learning models execute in-vehicle data processing in real-time and require huge amounts of computer power to run. As a result, when building in-vehicle applications, developers with limited computational resources find themselves too often confined to choosing between speed or accuracy. However, with safety hanging in the balance, the stakes are simply too high to compromise on either.
Unless speed and accuracy can both be sufficiently prioritized, advances in autonomous applications will remain in second gear. One way to overcome this obstacle? Well, car lovers know all about tuning; it’s all about getting the right mix of variables—speed, reliability—to achieve peak engine performance. The same principle can be applied toward optimizing deep learning models.
Driving into the deep end
The deep learning models embedded into ADAS systems process huge amounts of data collected from cameras and other sensors in the vehicle. With all that data, deep learning models empower autonomous vehicles (AVs) to “think”, or rather, process information almost instantaneously, in order to make the split-second decisions human drivers make on the road every day.
Each obstacle the automotive industry encounters is a catalyst for innovation
Picture a vehicle cruising down a street when suddenly a ball bounces onto the road. The vehicle has to process this input, predict the possible trajectory of a child running after it, and choose the safest course of action—brake, swerve, etc. Since the cost of error is so high, ADAS systems need to be incredibly fast and accurate. Neither can come at the expense of the other. But developers are struggling to deliver on both equally, despite advances in deep learning models and edge devices.
While some experts have suggested cloud processing as an alternative, this solution comes with its own risks. Connecting to distant third-party cloud-based resources to power split-second decision-making comes with the possibility of transmission delays, critical lags in ADAS functionality, and even data breaches, all of which jeopardise safety.
Instead, AV safety lies in empowering computer processing within the vehicle itself. To do so, the issue of computing constraints must be overcome, meaning models must be tailored specifically to the edge devices they’re running on. These smaller, more efficient models can help development teams unlock every bit of computational power that edge devices have to offer in order to achieve optimal speed and accuracy.
Smaller models, greater safety
The more automotive developers shrink and tailor the models inside automotive hardware, the closer the industry moves towards total vehicle autonomy. Right now, there are many ADAS safety capabilities that stand to benefit from more tailored models, such as object detection—computer vision systems that give AVs the potential to instantaneously recognise and react to objects on the road including hazards. With more efficient models, ADAS sensors can quickly process complex visual data in real-time to enable collision avoidance and automatic emergency braking.
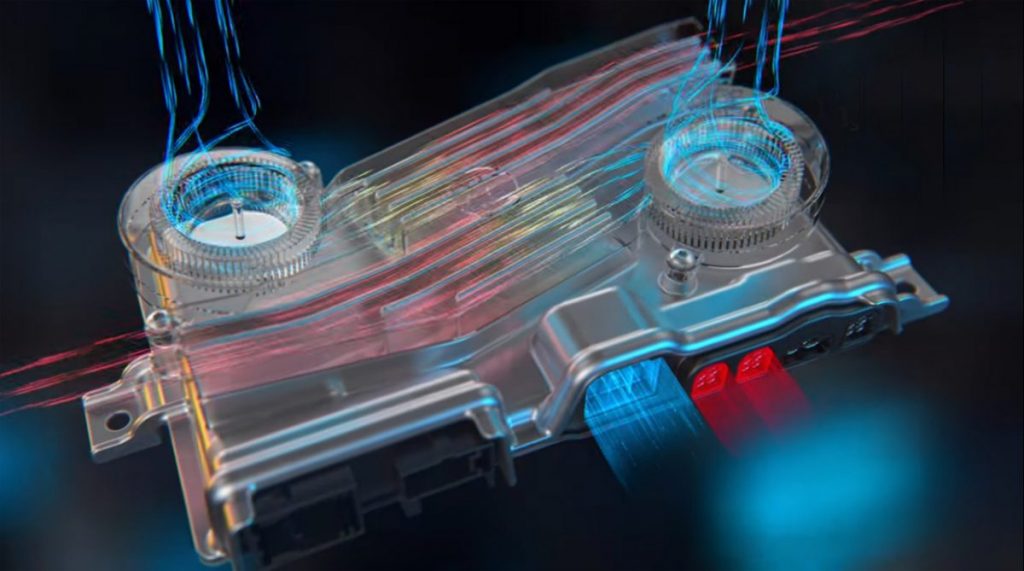
Smaller, efficient models also stand to enhance pose estimation. By analysing posture, head orientation and eye gaze of the driver, pose estimation models are poised to detect fatigue and distraction in real-time, preventing avoidable accidents by assessing attention and alertness of the person behind the wheel. If such signs are detected, this ADAS feature can eventually prompt vehicles to automatically assume command momentarily while the driver regains attention.
Localisation and mapping also receive critical enhancements through improved models. With the implementation of advanced remote sensing methods like LiDAR, ADAS systems can determine a vehicle’s position and map its surroundings. However, this feature requires a vast amount of accurate real-time data processing for autonomous applications.
Lane tracking is another of the several key ADAS capabilities that stand to improve from tailored models. Processing the visual data from lane markings is a continuous task that requires lightning-fast and accurate analysis for complete autonomous functionality. With these capacities, ADAS could take far more pressure off long-distance drivers by helping to keep vehicles from straying from their lanes.
The make and model
Many other automotive developments—in-vehicle infotainment, cyber security, energy management—are likely to focus their solutions around edge computing, where data processing occurs in the vehicle itself rather than being carried out in the cloud. Therefore, it becomes critical that deep learning models are not just accurate but also rapid and efficient to support the range of functions needed for AVs to become widely adopted.
Developers should aim for deep learning models that are compact and designed specifically for certain hardware architectures. To that end, dev teams must optimise efficiency rates so that the models are able to fully leverage available computational resources and memory usage of the edge devices they run on—ADAS, onboard computers, and telematic devices, among others.
In short, tailoring smaller models to specific hardware will help automotive developers cross the autonomous finish line.
About the author: Yonatan Geifman is Chief Executive and Co-founder of Deci